The AI chip market, which has been driving the recent AI boom, is largely dominated by NVIDIA. But now, Cerebras Systems, a rising venture, aims to disrupt this stronghold with a revolutionary AI chip that could redefine how we approach AI learning. In this article, we’ll explore Cerebras’ innovative technology, its potential to change the future of AI, and how it compares to NVIDIA’s established dominance.
- Cerebras is 20 times faster than NVIDIA in inference
- It rivals NVIDIA in the training process
- Cerebras may face challenges if NVIDIA advances its software
もくじ
- 1. Cerebras’ Ambition: Targeting Dominance in AI Learning Processes
- 2. Cerebras’ Strength: The Giant “Wafer Scale Engine” Chip
- 3. Exceptional Processing Speed and Programming Simplicity: Comparing to NVIDIA GPUs
- 4. Challenges of Wafer-Scale Chips: Yield and Software
- 5. NVIDIA’s Strength: The Ease of GPU Programming with CUDA
- 6. Cerebras’ Potential in Large Language Models (LLMs)
- 7. Who Benefits? The Target Audience for Cerebras and NVIDIA
- 8. Conclusion: The Future of AI Learning—Cerebras or NVIDIA?
1. Cerebras’ Ambition: Targeting Dominance in AI Learning Processes
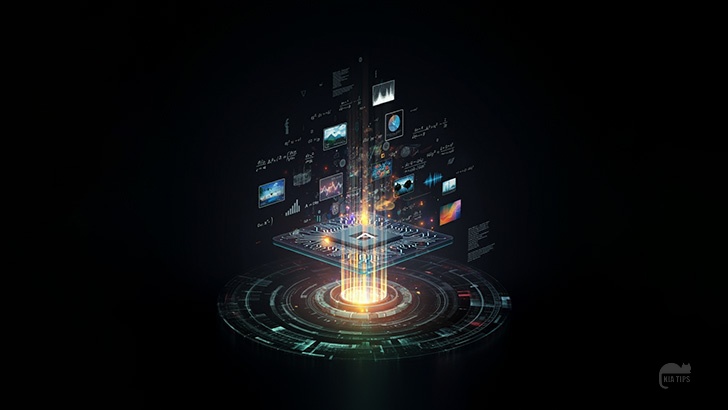
Cerebras is a uniquely ambitious startup among AI chip ventures, focusing specifically on capturing the market share of the AI “training process,” which NVIDIA currently dominates.
Supplement: What Are AI Training and Inference Processes?
AI development involves two major stages: the “training process” and the “inference process.”
- Training Process: This stage involves teaching the AI model with massive datasets. It’s like studying textbooks, requiring substantial computational power to process the data.
- Inference Process: Here, the AI model uses what it has learned to predict or make decisions based on new data, similar to applying knowledge in exams or real-world situations. Fast processing speed is key in this stage.
Recently, ventures like Groq have developed AI chips specifically for the inference process, competing with NVIDIA’s GPUs.
However, when it comes to the training process, NVIDIA’s GPUs hold an overwhelming share, creating a near-monopoly.
Cerebras aims to break this monopoly by developing a massive AI chip designed for the training process.
2. Cerebras’ Strength: The Giant “Wafer Scale Engine” Chip
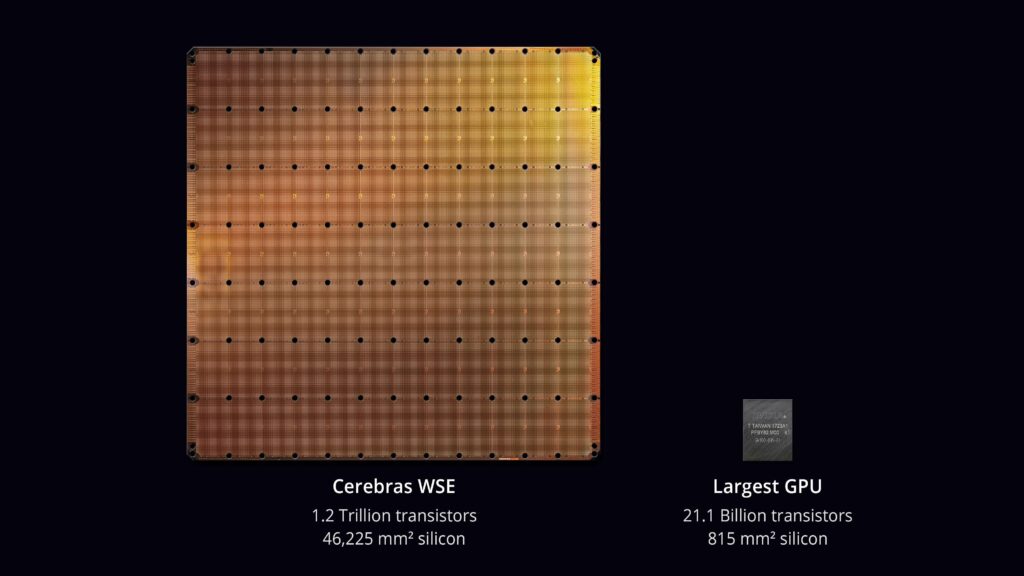
Cerebras’ standout feature is its massive chip, the “Wafer Scale Engine.”
While traditional chips are cut from wafers, Cerebras uses the entire wafer as the chip itself.
This is known as wafer scale, allowing for the inclusion of 900,000 processor cores, delivering computational power that was previously impossible with conventional chips.
3. Exceptional Processing Speed and Programming Simplicity: Comparing to NVIDIA GPUs
Cerebras’ chip boasts unparalleled processing speed in AI training, far surpassing NVIDIA’s latest GPU, the H100.
This is achieved by reducing inter-chip communication, eliminating the bottlenecks seen in traditional chip designs.
Compared to NVIDIA’s GPUs, which require communication between chips, Cerebras’ chip delivers 3,000 times faster communication speeds.
In inference, Cerebras also outperforms, being up to 20 times faster than NVIDIA’s GPUs, while reducing power consumption and overall operational costs.
Additionally, Cerebras has created a system where large clusters of its chips can be programmed as though they were a single unit, simplifying programming for models with massive parameters by a factor of 24 times compared to using traditional GPUs.
For instance, developing a large language model like GPT-4, which contains 1.7 trillion parameters, required over 240 developers, including 35 experts in distributed training and supercomputing.
Using Cerebras chips could potentially reduce the need for these specialists, optimizing development resources significantly.
4. Challenges of Wafer-Scale Chips: Yield and Software
While the innovation of wafer-scale chips is remarkable, larger chips tend to have lower yield (the proportion of functioning chips).
However, Cerebras has designed its chip to tolerate up to 5% of its 900,000 processor cores being defective, making the wafer-scale chip feasible.
Still, when AI models become too large to fit on a single chip, they must be split across multiple chips, requiring software to manage this division.
If NVIDIA develops similar software for smooth inter-chip communication, Cerebras’ advantage may be threatened.
5. NVIDIA’s Strength: The Ease of GPU Programming with CUDA
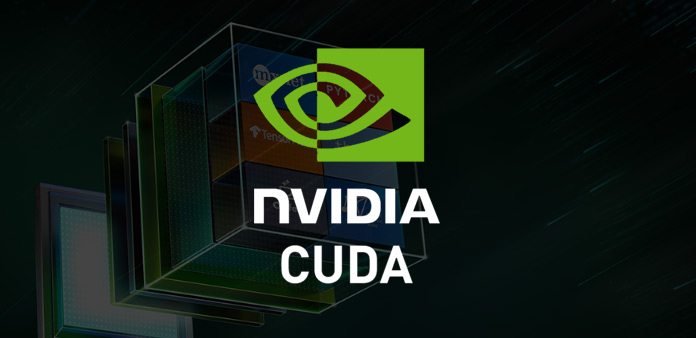
NVIDIA’s strength lies in its long-standing experience with GPU technology and the ease of programming provided by CUDA.
CUDA (Compute Unified Device Architecture) is a platform developed by NVIDIA to simplify parallel programming on GPUs.
With CUDA, developers can write programs in familiar languages like C++, fully utilizing GPU performance.
CUDA is widely adopted, with extensive software support, making NVIDIA’s GPUs versatile across a range of fields beyond AI, including gaming and scientific computing.
6. Cerebras’ Potential in Large Language Models (LLMs)
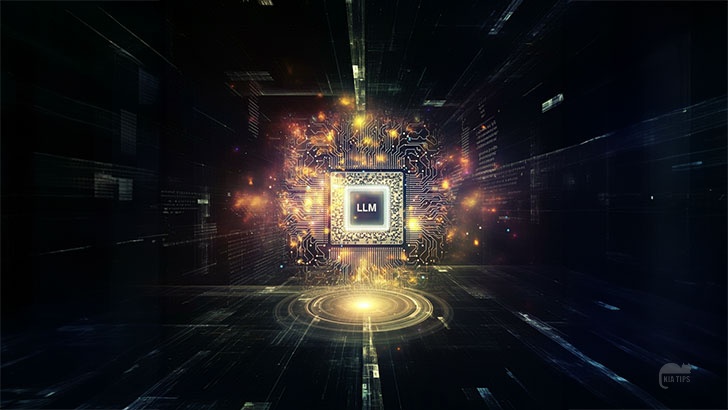
Cerebras’ chip is particularly promising for developing large language models (LLMs), which are gaining significant attention.
LLMs require massive datasets for training, and Cerebras’ chip, with its immense processing power and memory capacity, is ideal for such development. Traditionally, LLM training took substantial time, but Cerebras may drastically reduce this.
Supplement: What Are Large Language Models (LLMs)?
LLMs (Large Language Models) are AI models trained on vast amounts of text data for natural language processing.
These models can generate human-like text, answer questions, and perform translations. Recent LLMs such as ChatGPT o1-preview, Gemini, and Claude have brought major advancements in AI-driven natural language processing.
The key feature of LLMs is their vast number of parameters and the huge datasets used for training. For example, o1-preview has 200 billion parameters, requiring a powerful AI chip for training, like Cerebras’ Wafer Scale Engine.
7. Who Benefits? The Target Audience for Cerebras and NVIDIA
Who Should Choose Cerebras?
- Cutting-edge AI researchers: Cerebras is perfect for researchers working with large AI models, especially in fields like natural language processing and data-heavy sectors.
- Companies developing large AI models: Businesses aiming to use large AI models, such as high-precision chatbots or autonomous driving systems, will benefit from Cerebras’ processing power.
- AI developers seeking programming simplicity: Cerebras simplifies complex programming required for multi-GPU setups, allowing developers to focus on model design and algorithms.
Who Should Choose NVIDIA?
- Developers across multiple fields: NVIDIA’s GPUs are used not just in AI but also in gaming, simulations, and scientific computing. CUDA makes it easier to develop a wide range of applications.
- Cost-conscious developers: NVIDIA’s GPUs are expected to be more affordable, making them suitable for developers on a budget or for smaller projects.
- Developers utilizing a rich toolset: NVIDIA offers an extensive ecosystem of libraries and tools like CUDA, which streamline AI development and provide excellent support and resources.
8. Conclusion: The Future of AI Learning—Cerebras or NVIDIA?
Cerebras’ Wafer Scale Engine offers a transformative leap in AI learning processes, with its unparalleled speed and simplified programming capabilities. However, the company faces potential challenges from NVIDIA, especially if NVIDIA strengthens its software capabilities.
As the race for AI dominance heats up, it will be fascinating to see how Cerebras and NVIDIA continue to evolve. Could Cerebras’ innovation lead to a future where even small developers can participate in AI learning? Only time will tell, but it’s certain that the AI hardware landscape is on the verge of a major shift.
Source:Cerebras AI Day – Opening Keynote – Andrew Feldman – YouTube
Source:ow Cerebras is breaking the GPU bottleneck on AI inference
コメントする